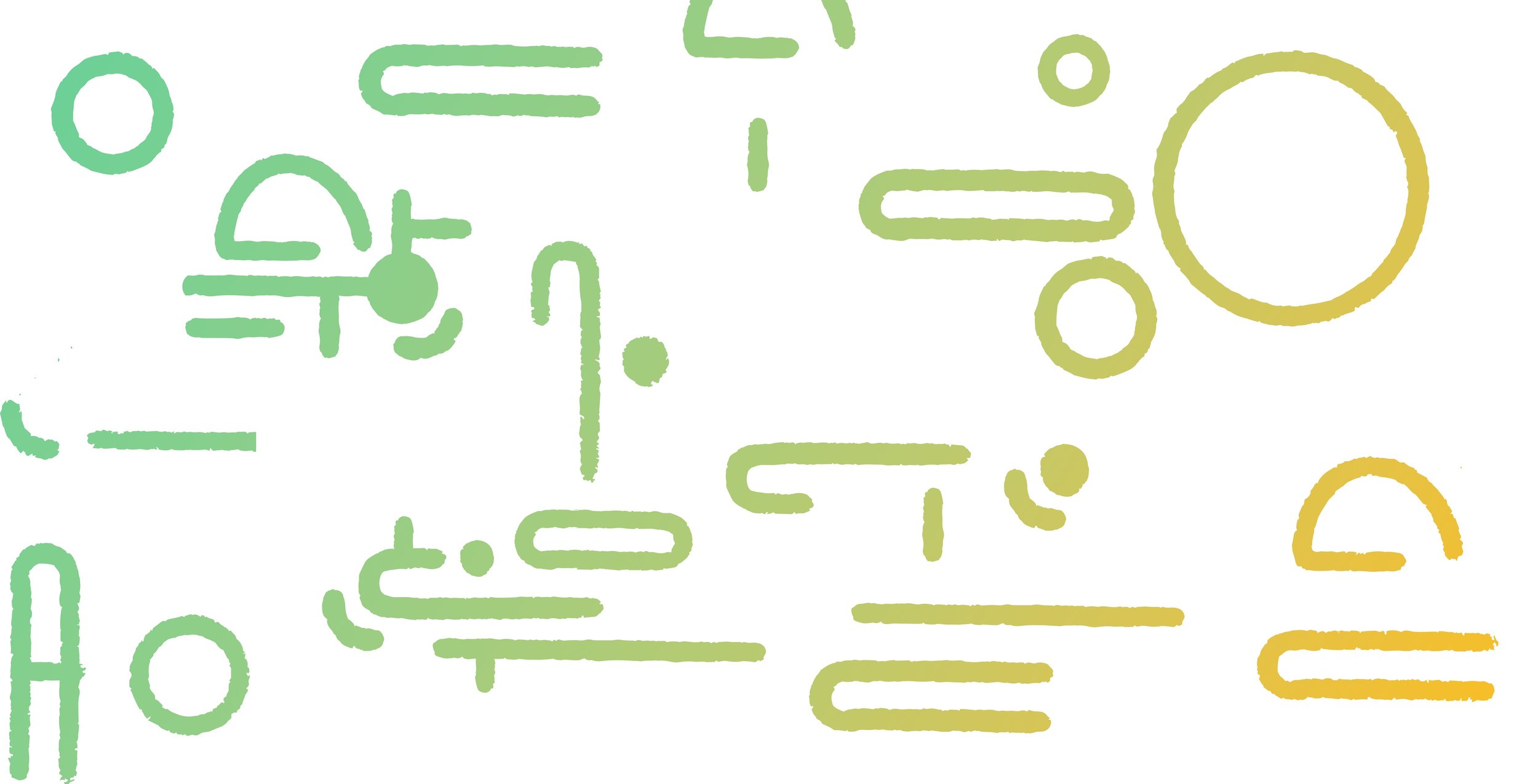
Creators, Put Your Content To Work
Contact us using the form below to let us know that you are interested in contributing to ArtFair’s data pool. We will reach out to discuss how your content can start working for you.
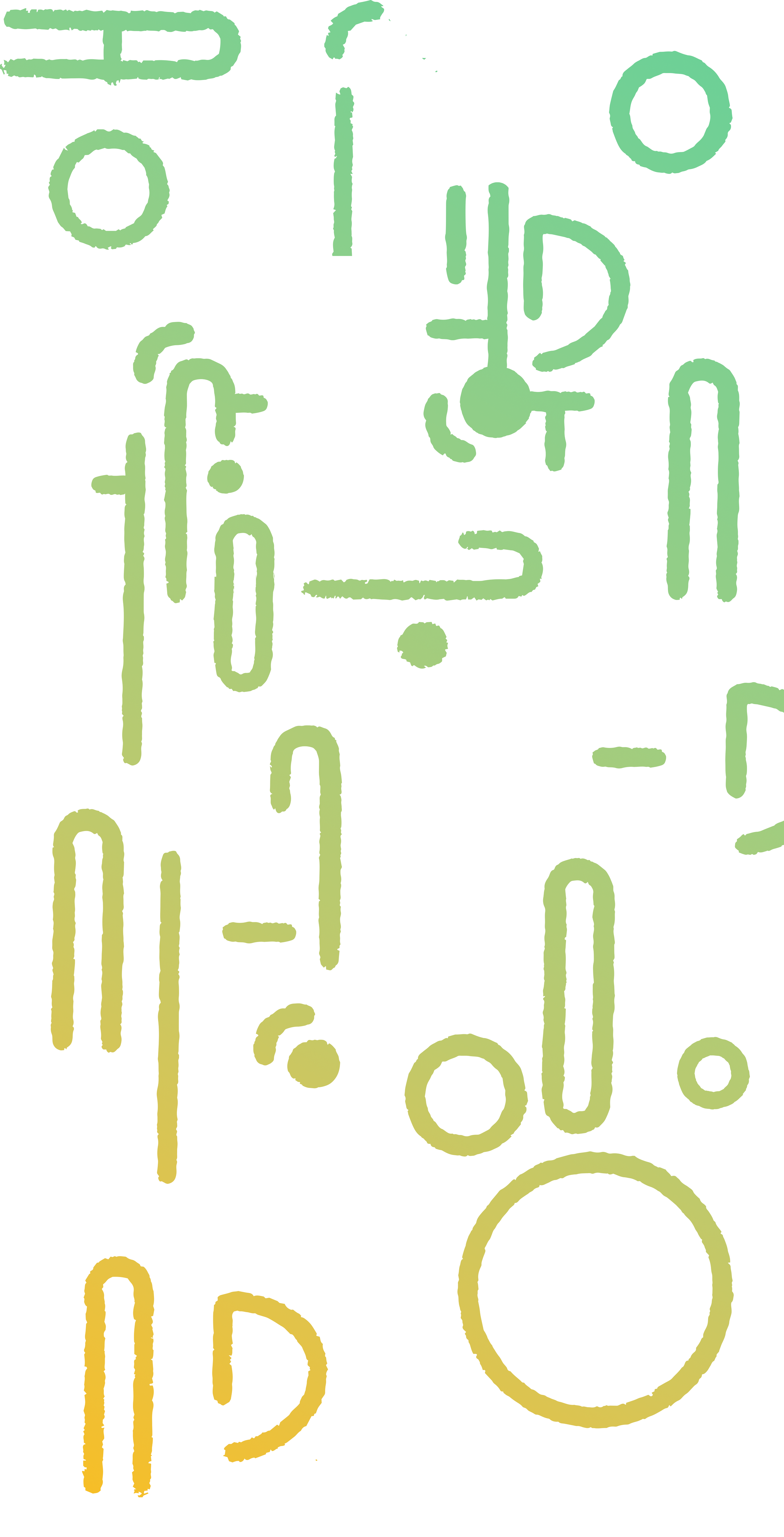
FAQs for Creators
-
Training data is a set of examples that show a computer program how to do something. After looking at enough examples, the program begins to infer how to succeed at a given task.
-
The exact way that an AI model learns from your content will vary by project. One common way that AI models learn from your content involves breaking it down into smaller units that it can recognize, called tokens. Different models can have their own ways of converting content excerpts into tokens. ArtFair’s transformation process breaks down your content into small pieces that are ready to begin the “tokenization” processes for a variety of AI training workflows. We also sort segments of your content into categories so that scientists can select the pieces that are most useful for their projects.
Other projects pair your content with labels and descriptions about what is in it to teach an AI how to recognize specific things. For example, labeling a video clip that shows a person talking to the camera with whether that person looks happy, sad, angry, or afraid can help teach an AI model to recognize facial expressions. Even if your content does not come with labels like that, it can still give scientists a starting point where they can add their own labels.
-
In short, a lot 😊. While some AI projects are looking to license as much human-generated content as possible for training, others are looking for targeted types of content to make their models smarter in specific areas. Here are a few examples of projects that our team has seen where your content could be useful:
Speech Models – Speech recognition models learn from listening to thousands of hours of spoken audio, paired with transcripts of what the people in that audio said. Even if it’s just a few friends chatting on a podcast or a video stream, exposing AI models to spoken content gives it more examples of how to understand human speech. The same types of content can even teach models to generate their own speech.
Large Language Models (“LLMs”) – Models in this family, like what you see in ChatGPT, learn how to write text by reading what people have written across a wide range of topics. Even if you are producing audio or video content, the transcripts and closed captions from that content produce useful text for LLMs to read. The ways people phrase things in spoken language can be very different from how they write in books, blogs, and articles, so your audio/video content can help these models learn how to chat and understand in a whole new way.
Video Generation – The latest generative AI models can produce videos based on a short text prompt. To make video that looks realistic, these models need to watch a lot of footage and learn from what they see. Video clips paired with summaries of what is happening in the clip are especially useful for this, but even videos without summaries can give scientists a starting point in their training workflows.
-
While some AI projects are looking to license as much human-generated content as possible for training, others are looking for targeted types of content to make their models smarter in specific areas. Whether any given scientist decides to license all, some, or none of your content for a given project will depend on 1) how unique your content is, 2) what topics are covered in it, and 3) how accurately you’ve labelled it.
Uniqueness – AI models learn better when they look at a wide range of different examples. For example, if an AI is trying to improve its speech recognition, it will learn best by listening to a wide variety of accents and unique words. If your content includes aspects that are underrepresented in our dataset, you might see it get licensed more frequently.
Topics – For scientists trying to improve their AI models’ fluency on a specific subject, they might want to license creative content that specifically discusses that topic. Those topics could be broad (e.g., sports, science, politics) or narrow (e.g., thoracic surgery, competitive Super Smash Bros., inventory management strategies in consumer electronics). Content that discusses certain topics might get licensed for AI training projects more often than others based on demand for those subjects.
Accuracy – When you upload your content to ArtFair’s data pool, it includes labels you applied to that content when you produced it, such as tags, alt-text, synopsis blurbs, and closed captions. These labels are especially powerful for certain types of AI training. We quality check the data you upload and score its accuracy when listing it on ArtFair’s marketplace. The more accurate your content’s labels are, the more likely your data will be to surface for a scientist looking to license it. We will surface any quality issues we find to you so that your work has the best chance of getting seen.